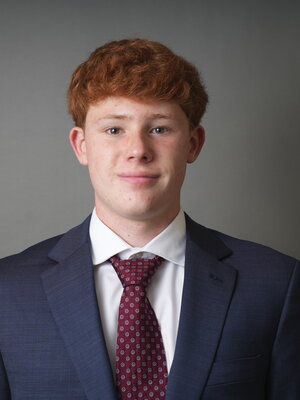
Daniel Carpenter is a junior Statistics & Computer Science major who is joining us at ATLAS for his first semester. Having heard about the program from department emails and newsletters, he joined hoping to get hands-on experience working with machine learning techniques and developing software that utilizes large language models (LLMs). Additionally, he saw the internship as an opportunity to help develop his technical skills while contributing to meaningful projects for ATLAS. At ATLAS, Daniel served as an Artificial Intelligence & Machine Learning Intern. Working as part of a collaborative team, he contributed to several projects, including completing the Kaggle Titanic data competition, building a course similarity web application using Natural Language Processing (NLP) models, and developing a research similarity web application that uses LLMs to categorize research topics. Through these experiences, Daniel gained a broad range of technical skills—mastering data cleaning, XGBoost, cluster analysis, and feature engineering. He also built experience working with NLP models and integrating popular LLM APIs into real applications. Weekly group meetings where he presented updates also helped him grow his time management and presentation skills. Daniel’s key takeaway from the internship is the importance of adaptability when working with real-world data. Unlike textbook examples, actual datasets are often messy and incomplete. Being able to clean data and work effectively with available features is critical for success. Looking ahead, Daniel plans to pursue a career as a data scientist or software engineer—continuing to explore the intersection of data, AI, and impactful problem-solving. But when he's not tinkering with data, he loves hiking and traveling!